"AI's carbon footprint is bigger than you think," warns MIT Technology Review. It’s a sobering assertion. As AI becomes increasingly integral to society, it’s crucial to weigh its costs and benefits carefully, ensuring we understand the full impact of its role. But before we all trade in our GPUs for abacuses (abaci?), let's take a moment to consider what this claim actually means.
Carbon Footprints
The article cites a study by researchers at the AI company Hugging Face, who calculated the CO2 emissions of various AI tasks and presented the graph below to illustrate their carbon costs.
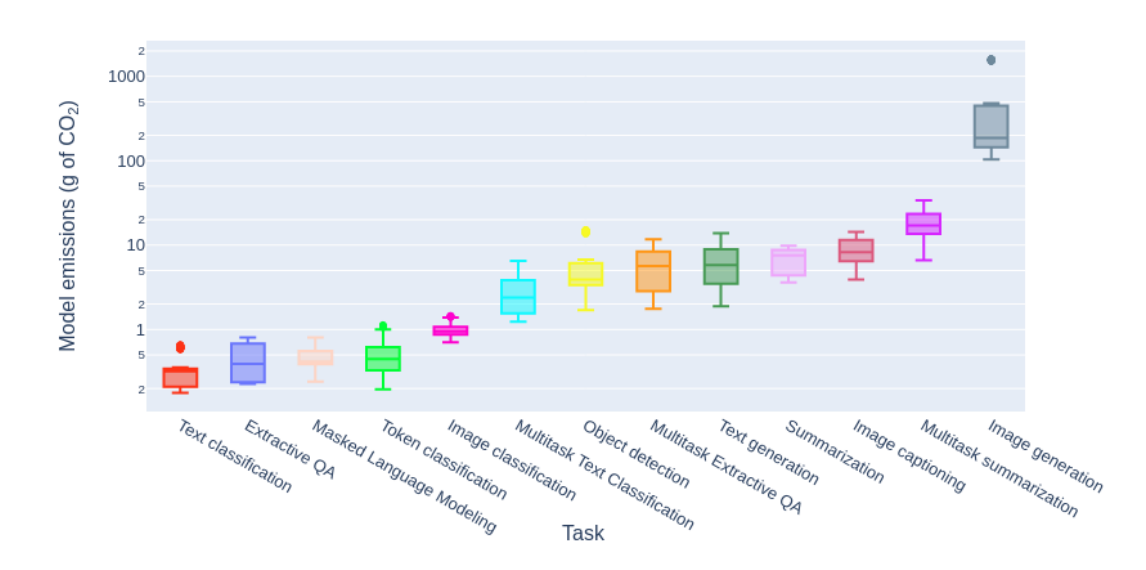
But this information, while interesting, doesn’t tell the full story. They measured the energy consumption of using AI to do things and compared it against… nothing. This makes AI look bad because there’s no context, no baseline. The lead author said in an interview, "Every time we query an AI model, it comes with a cost to the planet, and it's important to calculate that.” Well, sure—but you know what else comes with a cost to the planet? Everything. Literally everything. Every breath you take, every move you make (I'll be watching you), has a carbon footprint. The question isn't whether AI has a footprint; it's whether that footprint is larger or smaller than the alternatives.
Below is an image I generated with DALL-E 3 in a few seconds. Even if you don’t know much about AI models, compare the cost of a few seconds of computer time with the cost of driving to my local crafts store, buying some paint and canvases (which required carbon emissions to even appear on the shelf), and producing this. Or, if you want to compare generating this image with making it digitally, we could do that too. It’s a few seconds of human and computer time versus a few hours of human and computer time.
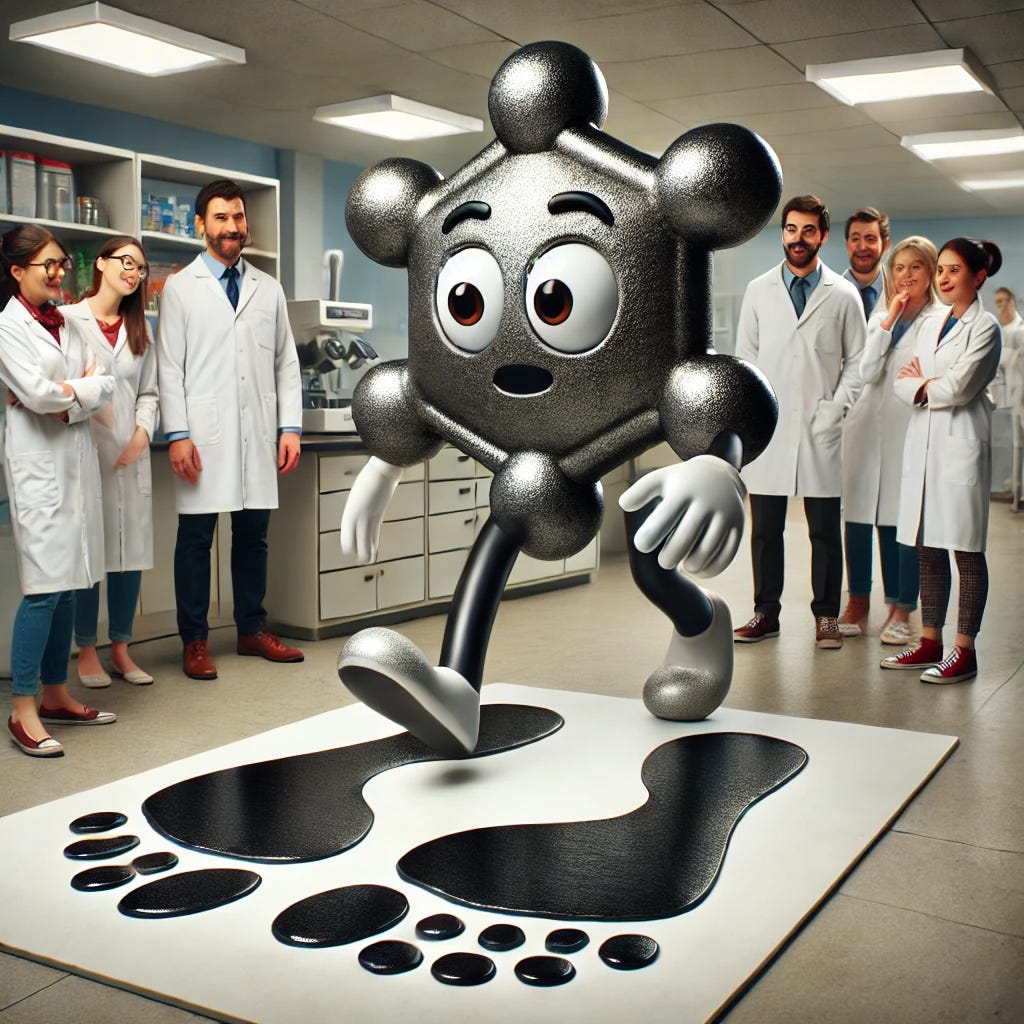
I thought I'd have to leave things there, relying largely on readers' intuition, but I actually found a study that did the correct comparison. They compared the carbon footprint of using AI to generate an image versus a human doing it. They found that “AI systems emit between 130 and 1500 times less [Carbon dioxide equivalent] CO2e per page of text generated compared to human writers, while AI illustration systems emit between 310 and 2900 times less CO2e per image than their human counterparts.”
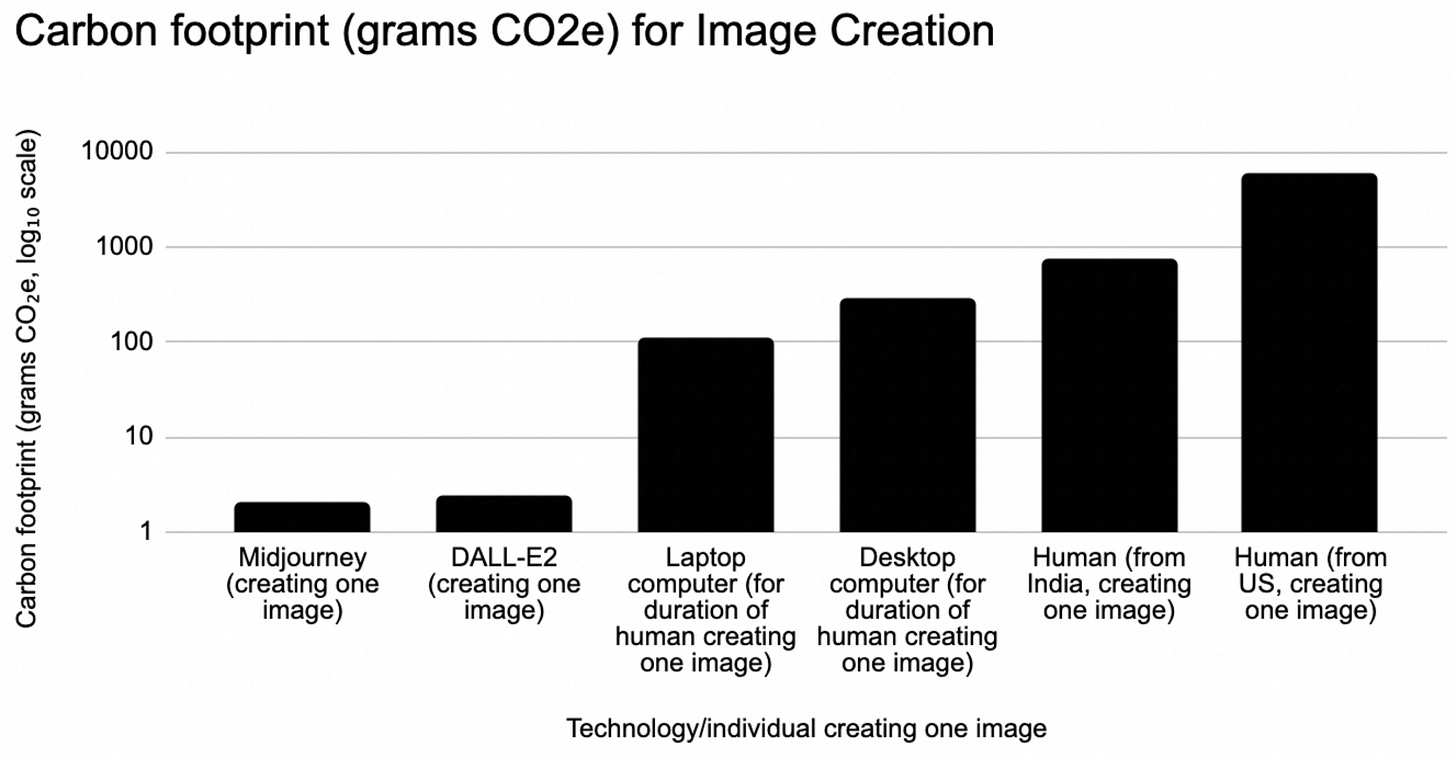
Anti-AI Bias
I think this speaks to a broader issue. There seems to be an anti-AI bias creeping into the public consciousness that, while understandable, distorts our perception of the technology. Some people appear to expect AI to solve problems while simultaneously having zero negative impact, a standard we don’t apply to anything else. No one asks about the carbon footprint of curing cancer, filming a movie, or, for that matter, baking artisanal sourdough bread. Yet, we scrutinize AI’s impact with a level of detail rarely applied to other activities.
The Moral Imperative for Self-Driving Cars
The consequences of this anti-AI bias are substantial. By holding AI to unrealistic standards, we risk delaying the adoption of beneficial technologies. Insisting on perfection might prevent the deployment of AI systems that could save lives or significantly improve quality of life, as we saw with the missed opportunity to use it in removing lead pipes in Flint, MI.
Perhaps the most important example of this is found in the case of self-driving cars. In an ideal scenario, self-driving cars will soon be, say, ten times safer than human drivers, and the public will gladly accept them. They will save lives and ultimately transform the transportation system into a safer and more efficient network.
But what if the technology falls short? What if it only becomes twice as safe and the technology stagnates around that level? Although the total number of traffic fatalities will decrease, the errors it makes will be different. People will notice the cars making mistakes that humans typically wouldn’t, which might lead them to oppose adopting the technology despite its potential to reduce fatalities.
It might not be immediately obvious why it's so important to adopt self-driving cars if they're only twice as safe as human drivers, so let me try to make the case. Road injuries are responsible for 2.14% of all deaths globally—more than malaria (1.18%), war and terrorism (0.23%), and homicide (0.70%) combined. This translates to approximately 1.35 million people dying on the world's roads each year. For people aged 5-29, traffic injuries are the leading cause of death. That's the scope of the moral imperative we're talking about. Reducing the number of traffic accidents by half would save more lives than ending all war, terrorism, and murder combined.
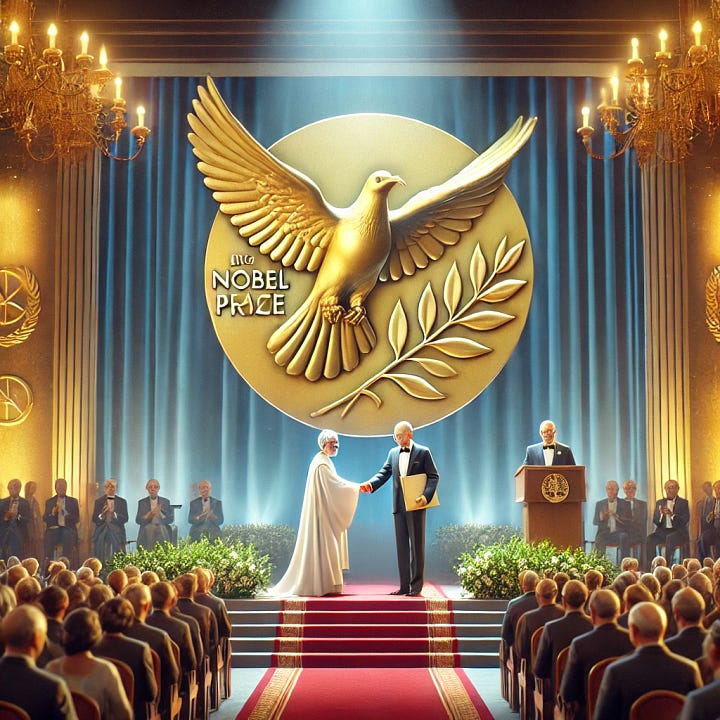
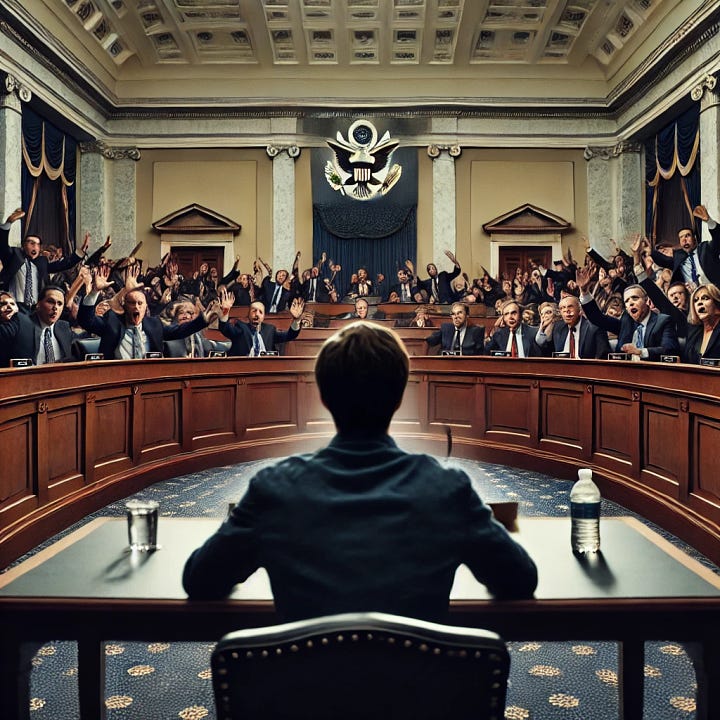
Even if self-driving cars are not perfect, their increased safety creates a moral imperative to push for their adoption. Over 40,000 people die in traffic accidents every year in the United States. Reducing this amount by 50% would save over 20,000 lives every year in the U.S. alone. We should stand on the side of cutting traffic deaths in half, on the side of those self-driving car companies, on the side of AI.
Balancing Anti-AI Bias and AI Risks
Anti-AI bias is, at its core, yet another human bias that impairs our thinking. It causes us to apply unreasonable standards to AI, expecting it to be a panacea. When we have concerns about AI, we should place those concerns in a broader perspective, weighing them against potential benefits and comparing them to the present, not the perfect. We shouldn’t expect it to fix all the ills of society. AI can do a lot, but AI isn't going to solve all our problems.
Nothing in this post is meant to suggest that we shouldn’t be concerned about how AI is used in our society. None of this is to say that AI can’t go wrong. Indeed, I think it can go horribly wrong.
But this changes nothing about the fact that anti-AI bias clouds our thinking. We need to think clearly—free from this bias—when considering both the tremendous benefits AI could bring to society and the potential dangers it may pose.