The water crisis in Flint, Michigan serves as a cautionary tale of what can happen when we fail to realize the potential of technology and data. This crisis began in 2014 when the city of Flint, Michigan, changed its water supply from Lake Huron to the Flint River. The Flint River's water had quality issues, including contamination with byproducts from corroded pipes, but the cost savings led government officials to make the change. The critical issue, however, was that the water from the Flint River was more corrosive than that from Lake Huron. When it flowed through the city's old lead-lined pipes, it leached lead into the water supply.
As is often the case with man-made disasters, many mistakes were made that caused this, but I won’t dwell on the details of who and what caused the problem. The crucial task soon became clear: the city needed to find all the lead and galvanized steel pipes and replace them with safe, copper pipes. In this post, I want to focus on how AI was employed in the search for these pipes, and how a lack of trust in it exacerbated an already grave situation, prolonging the crisis and causing unnecessary suffering.
Despite the terrible situation, there were promising results in the pipe inspection and removal process. A team of volunteers, backed by funding from Google, created a machine learning model to predict which homes were more likely to have pipes that needed to be replaced. The model used a combination of data sources, including historic water cards, a home's age and value, GPS coordinates, Census data, the previous year's replacement data, and water testing results, to predict if a home had hazardous pipes.
The model allowed the city to prioritize buildings that were most likely to have lead pipes. The approach proved to be effective. Out of the 8,833 pipes inspected, 6,228 contained lead, resulting in an accuracy rate of 70%. The model’s capability was evident, and its performance continued to improve as more data was added.
But not everyone favored the model. It would select some properties on a block, but not others. ‘You did my neighbor’s house and you didn’t do mine,’ residents complained to Mayor Karen Weaver. So when Flint signed a contract with engineering firm AECOM in 2018 to accelerate the program, Mayor Weaver “ordered the firm to investigate every house on selected blocks, without any particular methodology”, according to news source Water Online.
According to AECOM’s project manager, Alan Wong, getting citizens to rely on the model was a sticking point: “The program managers would have to tell people, ‘You’ll have to trust a computer model,’ Wong told The Atlantic. ‘The citizens are just not going to trust that.’”
Mayor Weaver's directive to spread the inspection across the entire city might seem like a fair approach. However, it ignored the fact that lead pipes were not equally distributed, and some houses within a block were much more likely to have lead or galvanized pipes than others. As reported by The Atlantic, “the lead was concentrated in a few areas, mostly in the older places in the core of the city, such as the Fifth Ward.”
There was also a concern that the model, even if it was good, wasn’t perfect. In November of 2018, MLive quoted Wong as saying: “The city stopped using the model because it has a 94 percent accuracy rate. The city wants 100 percent accuracy.” (The accuracy of the model seems to fluctuate based on precisely which period people are referring to, but the overall point remains the same.)
I don’t mean to imply that the decision to discard the model was completely due to fear of AI. The decision appears to be driven by a mix of political conveniences as well. For example, Mayor Weaver explained that the community's reaction was “less about a lack of trust in the model and more about a lack of trust in the government. ‘We didn’t want to use this method because we didn't want to miss anyone,’” she told Wired magazine.
There are also conflicting stories. In January of 2021, Wired reported, “Asked why AECOM didn’t use the model, Wong told city council members that his team was only offered a ‘heat map’ of potential lead pipe locations.” This is disputed by Eric Schwartz, one of the creators of the model, who told The Atlantic that he “sent five emails to Wong from January through May 2018, none of which was answered, and had offered its database, which consisted of individual lead-probability scores for every single address in the city.”
Like most decisions, this one was multifaceted, and it appears to have been influenced by an unfortunately heavy mix of politics. I still think that a broader societal distrust of AI played a role. The public and leadership were reluctant to rely entirely on a computer model, even though it had proven effective. This speaks to a climate of fear and skepticism surrounding AI technology. In a society with greater fluency in how algorithms function, perhaps they might have chosen a different path.
Instead of a targeted solution focused on areas where the lead pipes were likely to be, “Weaver also directed AECOM to spread work evenly throughout 10 zones of the city — so as not to favor one ward over another. That meant contractors couldn’t concentrate resources on all neighborhoods most likely relying on lead lines.”
Instead of the previous 70% hit rate for lead pipes, The Atlantic reports: “As of mid-December 2018, 10,531 properties had been explored and only 1,567 of those digs found lead pipes to replace. That’s a lead-pipe hit rate of just 15 percent, far below the 2017 mark.”
These numbers speak volumes about the efficiency of data-driven approaches. In a 2018 court filing, Schwartz estimated that between 4,964 and 6,119 homes were still afflicted with hazardous piping. A map created by the AI researchers (shown below) visualizes the impact of the new approach even more starkly.
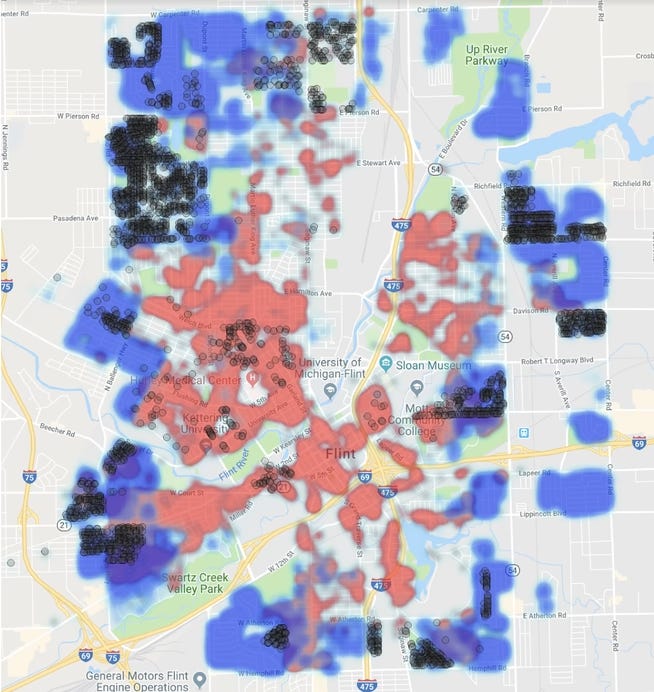
The Atlantic sums up the situation very well:
To take the most prominent example, the Fifth Ward is expected to have the most remaining lead. The University of Michigan model estimates that crews would find lead 80 percent of the time in that area. Yet from January to August 2018, AECOM contractors did the fewest excavations there, carrying out 163 excavations in the ward out of 3,774 total in the city. They found lead pipes in 156 of those digs—96 percent of them. Meanwhile, over the same time period in the Second Ward, 1,220 homes were investigated and lead was found in 46 of them, just a four percent hit rate. AECOM did the most digging in the two wards that Schwartz and Abernethy’s model predicted had the smallest percentage of lead pipes, and the results bore out the predictions of the model.
Simply continuing the 2017 program’s method might have pulled nearly all the remaining lead out of the city during 2018.
This story is a series of avoidable tragedies, where the decision to abandon a successful AI model led to unnecessary suffering. As I said, the reason for the change was multifaceted, but the following is undeniable: AI could have improved the situation, a decision was made not to use it, and more people were exposed to lead as a result.
Fortunately, it seems like a federal court ordered the city to start using the model again, though I haven’t been able to find detailed reporting on this since.
The Flint water crisis highlights a broader, often overlooked point: just as planes that don’t crash seldom make the news, futures that are never realized don't either. While the risks of AI are frequently discussed, we must also consider the positive impacts AI might bring. Part of the problem, as I’ve opined before, is that the term “AI” encompasses too much. This umbrella term covers vastly different technologies, from straightforward algorithms like XGBoost, used for identifying hazardous pipes, to complex large language models (LLMs) that we do not fully understand and could be dangerous. While some AI applications deserve more scrutiny, others receive undue criticism.
The possibility of a better future, and the question of whether we will seize it, will be a recurring one throughout the coming decades. I sometimes wonder what we’re missing and what we could gain by thinking more optimistically about AI. But these boundless possibilities exist only if we don't limit them. The promise of AI extends into virtually every facet of our existence, holding the potential to bring about profound changes.
The march of artificial intelligence will inevitably bring us to a crossroads where we must balance skepticism against the promise of progress. If we measure AI against perfection and let fear rule our approach we risk losing a wellspring of potential benefits. Even an imperfect AI system can present significant improvements over existing solutions. This isn't about being reckless; it's about being visionary and recognizing the tangible improvements AI can bring to human existence.