Imagine you're a triage nurse in a war-torn country. The air raid sirens have just fallen silent, and the first wave of casualties is streaming into your makeshift hospital. To your left, a soldier with a severe head wound; to your right, a child with shrapnel in her leg; straight ahead, an elderly man clutching his chest. You have seconds to decide who gets the last dose of morphine, who gets rushed to surgery, and who gets told to wait.
How do you decide?
If you're like most people, your gut is already churning at the mere thought of having to make such a decision. But you don't have the luxury of throwing up your hands and saying, "It's too complex! Each life is infinitely precious!" In those seconds, you will make a decision. You will, in effect, assign values to these lives and their suffering. You will quantify the unquantifiable.
This scenario, while fantastical, illustrates a criticism often leveled at the effective altruism (EA) movement. Critics argue that EA's approach amounts to calculating moral decisions as if on a spreadsheet, reducing complex human experiences and values to mere numbers. They protest that you can’t put a number on human life or the impact of a charity because not everything can be quantified.
It's a compelling argument that resonates with our intuitive sense that some things are beyond measure. After all, how can you possibly assign a numerical value to the laugh of a child, the inspiration of a great work of art, or the comfort of a loved one's embrace?
But we do it anyway. We do it because we have to, because, in a world of limited resources, we're all triage nurses in our own way. Every time we choose to spend money on ourselves instead of others, every time we decide to donate to one charity over another, every time we allocate our time and energy to one cause over another, we are implicitly making these calculations.
The question isn't whether we should quantify the unquantifiable, but whether we should do it explicitly, systematically, and with our eyes wide open to the limitations and pitfalls of such an approach. Or will we pretend we don’t need to think about it, making choices with little thought and ignoring the unseen trade-offs lurking beneath each choice?
The Nature of Reduction and Quantification
"You can't compare apples and oranges."
It's a phrase we've all heard, usually trotted out when someone's trying to shut down an argument comparing two things. It's meant to be a conversation-ender, a pithy way of saying, "These things are too different to be meaningfully compared."
But we compare apples and oranges all the time. And not just metaphorically.
Say you're walking to work and can only grab one piece of fruit. In that moment, if you want to eat, you’re literally comparing an apple to an orange. And in doing so, you've just placed these two fundamentally different fruits on a single axis: "the best option to take with me right now." You're not comparing their DNA or their photosynthesis processes. You're comparing their ability to solve your immediate problem.
If you're planning to eat while you walk, the apple wins—you don’t want to have to peel something while power-walking down a busy sidewalk. But if you want an extra dose of vitamin C, the orange wins.
The point is that we are constantly creating these invisible axes of comparison. We're taking disparate objects, concepts, or choices and lining them up on a single axis that matters for our current situation. In doing so, we're creating abstract representations or models of these things, simplifying their complexity into something manageable. We're all constantly creating single-dimensional projections of our multi-dimensional world.
All Models Are Wrong
This doesn’t mean the representations are perfect. For example, imagine you start with three apples, add three more, eat four, then add one. Now you’re back to three apples again, exactly where you started. But, in reality, you’re not exactly where you started because no two apples are identical, and you don’t have all the same apples.
This doesn't imply that the mathematical model “doesn’t work”; it simply means its application has limits. It counts the apples accurately but doesn't account for their uniqueness.
Does this mean our mathematical model is wrong? That it "doesn't work"? Not at all. It just means its application has limits. It counts the apples accurately but doesn't account for their uniqueness.
This limited nature isn't a bug in our model; it's a feature of all models. As the statistician George Box famously said, "All models are wrong, but some are useful."
The key is to remember that these models are tools, not truths. They're meant to guide our thinking, not replace it.
Money as a Universal Quantifier
We've established that comparing apples and oranges isn't just possible, but something we do all the time. We put them on a single axis—be it taste, convenience, or nutritional value. But comparison is just the first step. To truly harness the power of this thinking, we need to take it further. We need to quantify.
Quantification isn't just about slapping numbers on things for the sake of it. It's a powerful tool that transforms vague preferences into precise, actionable information. It provides precision, allowing us to express the degree of our preferences, and enables comparability, making it possible to compare anything with a number. Quantifying also supports aggregation and clearer communication.
In everyday life, money stands out as one of the most practical and widespread tools of quantification, acting as a universal metric for comparing a vast range of goods and services. To illustrate this, let's consider two companies: Alfred's Apples and Bonnie's Bananas, both seeking loans from a bank. Alfred's Apples dominates the lucrative apple market, while Bonnie's Bananas is a major player in the less profitable banana market. Comparing these companies for loan purposes is challenging due to their different products. This is where money becomes a crucial tool.
By quantifying the value of Alfred's Apples at $100 million and Bonnie's Bananas at $50 million, we suddenly have a common scale. We can compare them, make decisions, and allocate resources.
But it gets even better. Once these companies go public, their stock prices become a real-time, continuously updated measure of their value. Every piece of news, every quarterly report, every rumor about a new apple flavor or banana shape is instantly processed by millions of investors. Their valuations reflect the collective judgment of all investors, condensed into a single number per company.
The market is one of the most efficient information processing systems to have ever existed on the planet. It's like a giant, global brain that's constantly thinking about the relative value of every publicly traded company. Is it perfect? Absolutely not. But it's a pretty impressive tool for aggregating information and opinions from countless sources into a single, easy-to-compare number.
That’s all well and good for apples and bananas, but what about the important things, like human lives? Again, the exact same thing is true: quantification is a tool that can help us answer questions, but it has limits.
Quantifying Human Life
Government agencies often face challenging decisions where they must weigh the cost against the potential for saving lives. To facilitate this, they assign a monetary value to a statistical human life. For example, the EPA uses a value of $7.4 million (in 2006 dollars), while the Department of Transportation uses $9.1 million. The exact figure isn't as crucial as the fact that there is a number. This allows agencies to evaluate life-saving investments more systematically. This allows them to look at every potentially lifesaving investment and say, “Find out how many lives it would save. Find out how much it would cost. Now find the cost per life and let’s look in more detail at the proposals where the cost is below $10 million.” This approach provides a practical framework for assessing the cost-effectiveness of various safety measures.
This does not mean that “a human life is worth $9.1 million.” No one is saying that. They are, however, saying that quantifying it as such is a useful tool. The EPA’s website that lists the $7.4 million number, in anticipation of criticism, makes this explicit:
The EPA does not place a dollar value on individual lives. Rather, when conducting a benefit-cost analysis of new environmental policies, the Agency uses estimates of how much people are willing to pay for small reductions in their risks of dying from adverse health conditions that may be caused by environmental pollution.
While some may object to the practice of assigning a monetary value to human life, in practical terms, we implicitly understand that such a valuation is inevitable. The belief that human life is priceless is a fundamental ethical stance, yet it conflicts with practical limits in decision-making. Consider an extreme scenario: would it be feasible to spend a trillion dollars to save a single life? Most would say no. Conversely, spending one dollar to save a life would be an unequivocal yes. This suggests that the value of a life, though immeasurable in moral terms, falls somewhere between these two extremes in practical scenarios.
Caveats and clarifications:
Yes, there’s more to life than being alive.
Yes, some improvements are worthwhile but don’t save lives.
No, I would not be willing to sell my life for $10 million.
Quantifying the value of a statistical life doesn’t dispute any of these statements. It's just a framework for making decisions.
Quantification in Moral Philosophy
OK, but how do you quantify questions of moral goodness? Philosophers have long grappled with this issue, yet a definitive answer remains elusive. This doesn’t have to paralyze decision-making. For example, let’s compare the value of preventing blindness versus giving a guide dog to a blind person. My guess is most people would say preventing blindness is more valuable. Some might believe curing blindness is twice as valuable, others might value it ten times more, while some see both actions as equally important. Despite the disagreement, we can still ask each person how they would rank a charity that trained guide dogs and one that cured blindness. According to philosopher Peter Singer’s Ted Talk, “It costs about 40,000 dollars to train a guide dog and train the recipient so that the guide dog can be an effective help to a blind person. It costs somewhere between 20 and 50 dollars to [prevent] a blind person in a developing country if they have trachoma.”
Even if you think these numbers are off, or you think training a guide dog is 10 times more valuable than curing blindness, you would still conclude that the charity preventing blindness is a more effective investment. This example illustrates that, despite disagreements in moral valuation, we can still use quantifiable data to inform our decisions about charitable effectiveness.
Just to be absolutely clear, my argument isn't that providing guide dogs to blind people is not valuable. If it seems like I'm suggesting that, then the point has been misunderstood. The comparison between preventing blindness and training guide dogs was to illustrate the complexities and considerations in evaluating the effectiveness of different charitable actions. It's not about devaluing one noble cause in favor of another, but about exploring how we can make informed decisions with limited resources.
By putting numbers to these scenarios, we can start to make more informed decisions. We can start to answer questions like:
If I have $X to donate, how can I help the most people?
Is it better to solve one big problem or many small ones?
How do we weigh immediate relief against long-term development?
Quantification in moral philosophy isn't about reducing human experiences to numbers. It's about using numbers as a tool to guide our moral decision-making. It's about trying to do the most good we can, rather than just feeling good about doing something.
Limitations
It is, of course, important to recognize the limitations of quantification. Take the earlier example of Alfred's Apples and Bonnie's Bananas, where Alfred's company is valued at twice that of Bonnie's. This valuation doesn’t mean that splitting Alfred’s company in half would create two entities comparable to Bonnie’s Bananas, even though they might be monetarily “equivalent”. That would be extending quantification beyond its logical and useful scope.
Similarly, when quantifying ethical decisions, it's important to note that not all mathematical principles directly translate. For instance, performing a good deed today doesn't provide a moral license to commit a smaller bad deed in the future. This is another case where a literal interpretation of quantification can be misleading.
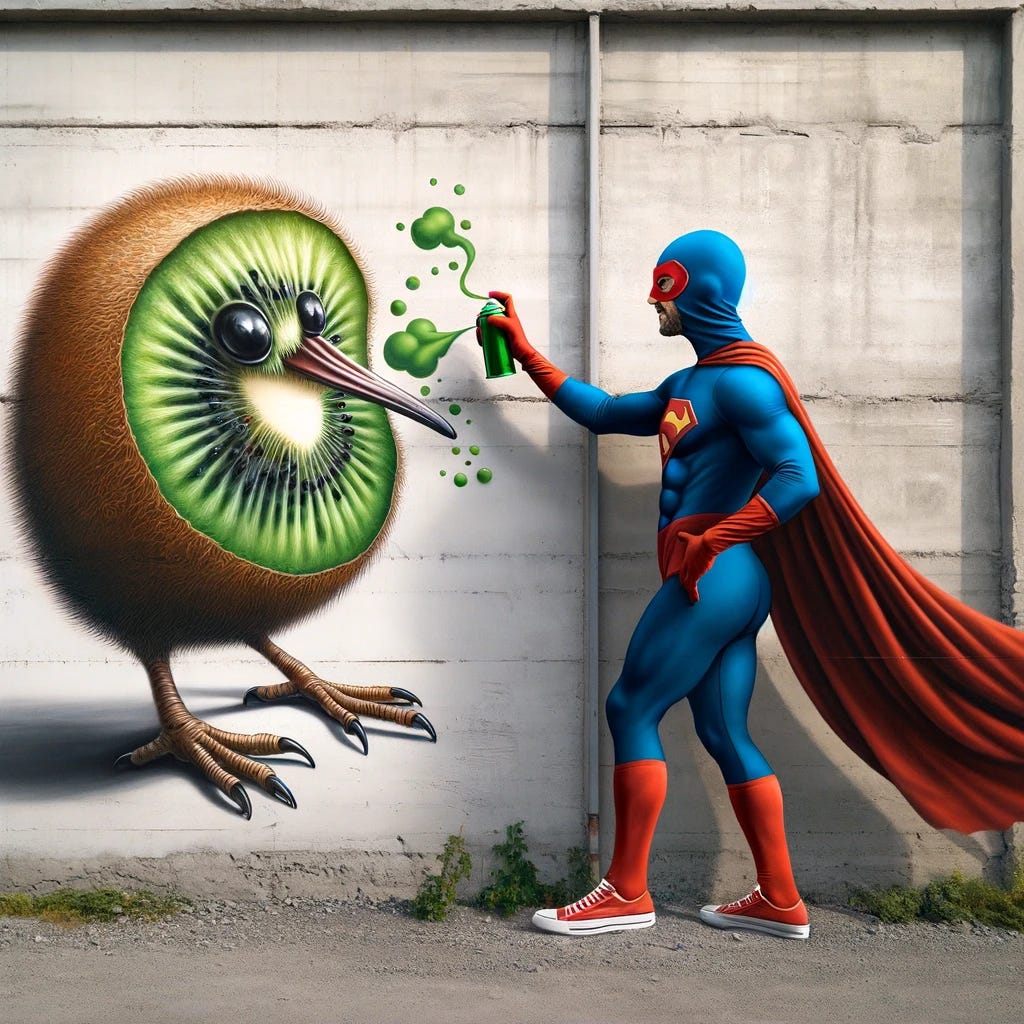
The key point is not that quantification provides perfect answers, but that it can be a useful aid in decision-making. Its value lies in utility rather than absolute truth. A human life isn't worth $10 million. There's no such thing as -1 apples. If you accidentally donated more money than you meant to a charity, it doesn’t mean you’re now allowed to go knock over an old lady crossing the street to balance things out. No one is advocating for such literal interpretations.
Conclusion
Quantification in moral reasoning forces us to confront uncomfortable truths, make difficult comparisons, and sometimes reach conclusions that feel intuitively wrong. It's the ethical equivalent of watching sausage being made—useful, but not always pleasant.
But in a world of limited resources and endless needs, we don't have the luxury of avoiding these difficult questions. We make trade-offs every day, whether we acknowledge them or not. Quantification doesn't create these trade-offs; it just brings them into the light where we can examine them.
Yes, there's an emotional discomfort that comes with reducing complex moral issues to numbers. It can feel cold, impersonal, even dehumanizing. But consider the alternative: making decisions based solely on gut feelings, unchallenged assumptions, or whoever shouts the loudest. Is that really more humane?
The practical necessity of quantification doesn't make it any more comfortable, but it does make it important to get right. And getting it right means using it wisely, which brings us to our call for a balanced approach:
Use quantification where it adds clarity and aids decision-making.
Be willing to look beyond the numbers to context, nuance, and qualitative factors.
Regularly question and update our methods of quantification.
Be transparent about the assumptions and limitations of our quantitative models.
Use quantification to inform moral decisions, not to make them for us.